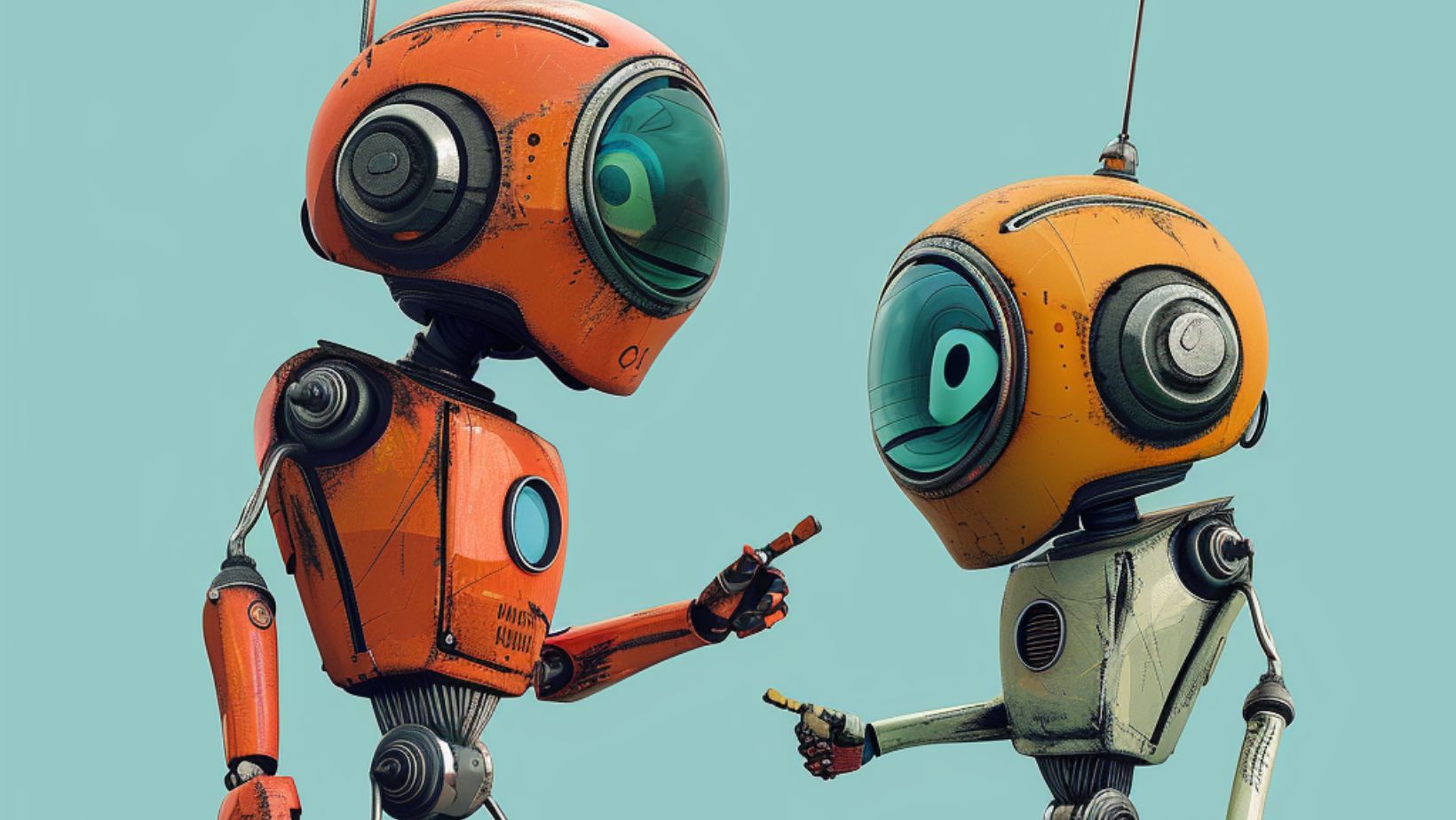
As AI usage increases across industries, it drives rapid technological evolution. New concepts and ideas emerge, transforming into models that can offer a competitive edge. This is why innovation-focused businesses keep a close eye on developments related to large action models (LAMs) and their potential applications.
LAMs are touted as the next major technological disruption, promising to reshape the future. However, information about them is limited, leaving many unanswered questions and areas of uncertainty. Are LAMs really as revolutionary as they appear?
Given the complexity of artificial intelligence, it is crucial to thoroughly investigate any new advancements before taking action. This article aims to shed light on the unknowns and dispel the myths surrounding large action models.
Large Action Models: Debunking The Myth
The true answer to “What is a LAM?” is quite straightforward — large action models don’t actually exist.
The term LAM emerged in the tech community when the AI startup Rabbit began marketing their AI device to a B2C audience. Rabbit’s campaigns promote their product, R1, which operates on an innovative Rabbit OS. This system is allegedly powered by a new form of artificial intelligence designed to make complex decisions and function as an intelligent assistant for everyday tasks. Rabbit OS can assist users with activities such as ordering groceries, reserving restaurant tables, playing music on request, and sending messages.
The first red flag regarding large action models (LAMs) is that discussions about them often highlight their potential benefits across various industries rather than showcasing concrete achievements or current implementations. There are no established metrics, a market to monitor, or widely recognized use cases for LAMs yet.
Although this lack of information can be attributed to LAMs being a nascent trend, decision-makers should approach with caution, critically evaluating the hype and focusing on verified facts.
For example, there are several facts about LAMs that executives should be aware of:
- Adoption misconceptions: There is a belief that enterprises are already widely adopting LAMs. However, currently, there are no real case studies of LAM being leveraged across B2B or B2C sectors.
- Current use and availability: After being released, Rabbit OS gathered a number of negative feedbacks, accusing developers of dishonest marketing and misleading descriptions. This situation challenges the notion of LAMs’ industry-wide application and their dominance over large language models.
- Lack of actual proof of value: The idea that large action models (LAMs) can execute critical tasks demands careful examination. While LAMs theoretically have the potential to handle complex and important functions, theory alone is insufficient to convince stakeholders—and rightly so. Even the feasibility of LAMs performing simple tasks is still under investigation, making it essential to distinguish between expectations and reality.
- No tangible impact across industries and enterprises: As the first use case of a large action model remains forthcoming and primarily consumer-focused, it is premature to discuss any industry-wide benefits. The technology has yet to prove its efficacy and demonstrate its advantages. Only through rigorous testing and user feedback can its true value and competitive edge be determined. While artificial intelligence is undoubtedly a transformative force with a significant global impact, it is crucial to focus on its proven capabilities rather than speculative claims that have not yet been substantiated.
Thus, while the concept behind LAMs is incredibly promising, it’s not actually real. Therefore, it’s crucial for executives to sift through optimistic projections and base decisions on tangible evidence and practical demonstrations.
For instance, the product promoted as LAM was revealed to be a ChatGPT using a number of hard-coded scripts. This reveals that business leaders and audiences interested in technology don’t have a full understanding of what large language models are capable of and how much value they can deliver.
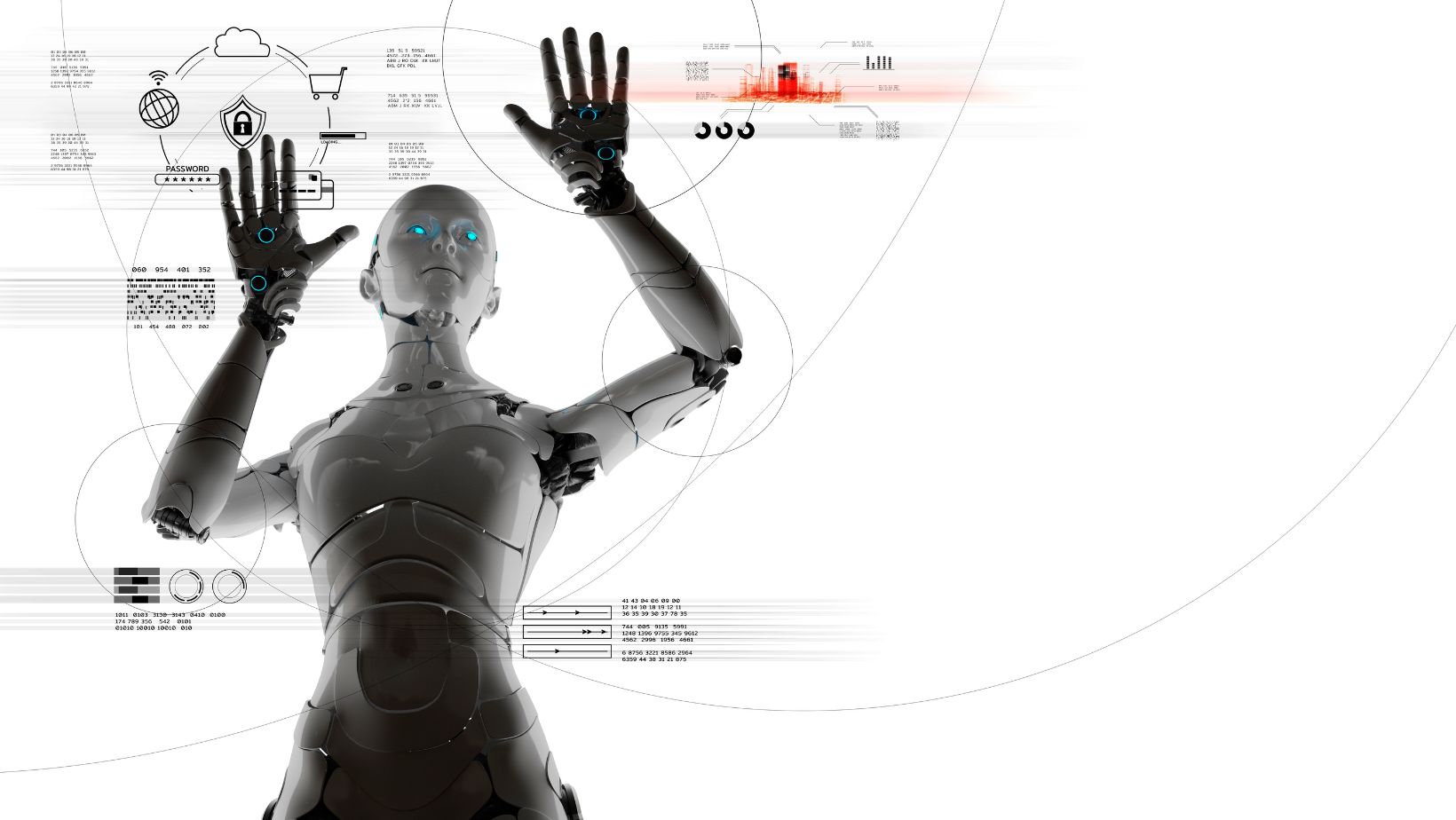
Due to this, it makes sense to explore available options that have proven themselves, can perform the same tasks LAMs are claimed to perform, and have a solid and easy-to-understand framework. For instance, large language model agents or LLM agents have been widely used for complex task management, driving realistic results and bringing measurable value.
Understanding Large Language Model Agents
Large Language Model agents are AI systems built upon the foundation of LLMs but are designed to operate as autonomous entities capable of performing specific tasks and interacting with users. Unlike traditional LLMs that primarily generate text based on input prompts, LLM agents incorporate additional layers of functionality, such as decision-making, context management, and real-time interaction capabilities. These agents can process vast amounts of data, understand context, and execute commands, making them versatile tools for various applications.
Key components of large Language model agents
-
Natural Language Processing (NLP)
At the core of LLM agents is their ability to understand and generate natural language, allowing them to interpret user queries and provide coherent, contextually relevant responses.
-
Context Management
LLM agents maintain context over extended interactions, enabling them to remember past interactions, understand nuances, and provide more accurate and personalized responses.
-
Decision-Making Algorithms
These agents incorporate decision-making frameworks that allow them to analyze options, weigh consequences, and choose the best course of action based on the information available.
-
Task Execution
Beyond generating text, LLM agents can perform tasks such as scheduling appointments, conducting research, managing emails, and more by integrating with other software systems and tools.
-
Learning And Adaptation
LLM agents continuously learn from interactions, adapting to user preferences and improving their performance over time through machine learning techniques.
Applications Of LLM Agents
Unlike large action models, LLM agents are increasingly being applied across various industries, revolutionizing how organizations interact with technology and streamline operations. The versatility of LLM agents allows them to optimize workflows, enhance productivity, and facilitate better decision-making across diverse sectors, making them an invaluable asset in the modern digital landscape.
-
Customer Support
LLM agents are revolutionizing customer service by providing instant, accurate responses to customer inquiries, resolving issues, and handling multiple interactions simultaneously, thus improving customer satisfaction and reducing operational costs.
-
Personal Assistants
As personal assistants, LLM agents manage schedules, send reminders, book appointments, and perform a variety of personal management tasks, enhancing productivity and organization.
-
Content Creation
LLM agents assist in generating high-quality content for blogs, marketing materials, and social media, significantly reducing the time and effort required for content production.
-
Healthcare
In healthcare, LLM agents support doctors and patients by providing medical information, scheduling appointments, sending reminders for medication, and even offering preliminary diagnostics based on symptoms.
-
Education
LLM agents serve as tutors, providing personalized learning experiences, answering questions, and helping students with assignments, thereby enhancing the educational experience.
-
Business Operations
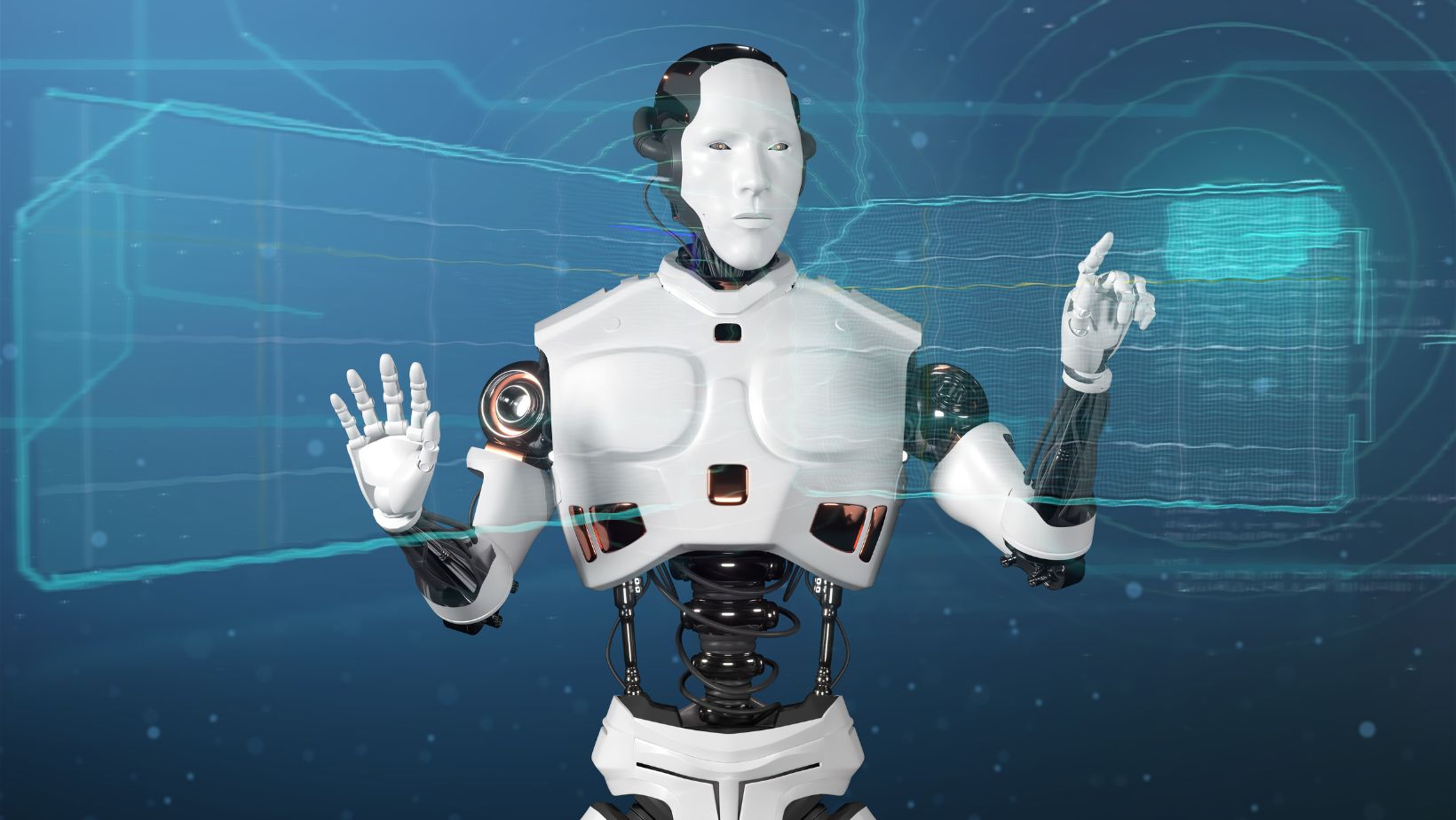
Businesses leverage LLM agents for tasks such as data analysis, report generation, market research, and managing communications, thereby streamlining operations and boosting efficiency.
Future Prospects
The future of LLM agents is promising, with ongoing advancements poised to enhance their capabilities further. Potential developments include:
-
Enhanced Multimodal Interactions
Future LLM agents may integrate capabilities to process and generate not just text but also images, videos, and audio, leading to more comprehensive and interactive user experiences.
-
Improved Emotional Intelligence
Enhancing the emotional intelligence of LLM agents will enable them to better understand and respond to human emotions, improving the quality of interactions and user satisfaction.
-
Domain-Specific Expertise
Developing LLM agents with specialized knowledge in specific domains such as law, medicine, or engineering will enable them to provide more accurate and expert-level assistance.
-
Seamless Integration
Advancements in APIs and interoperability standards will facilitate smoother integration of LLM agents with various software systems and platforms, expanding their utility across different industries.
Large Language Model agents represent a significant leap forward in the capabilities of AI, offering dynamic, intelligent, and versatile solutions for a wide range of applications. As technology continues to advance, these agents will become increasingly sophisticated, enhancing their ability to interact with users, perform complex tasks, and drive efficiencies across industries. Embracing the potential of LLM agents will enable organizations and individuals to unlock new levels of productivity, creativity, and innovation.
Conclusion
While large action models don’t currently exist, the LAM case provides a valuable lesson on the importance of separating marketing hype from facts and reality. They also show how important it is to look at the currently available solutions such as LLM agents and explore the full extent of their capabilities.
Nevertheless, this article doesn’t imply that more complex and sophisticated versions of AI systems will never enter the market. Initiatives aimed at developing autonomous AI systems for solving complex problems and making decisions will undoubtedly emerge.
However, innovators must also consider persistent challenges: hardware limitations and energy consumption remain significant obstacles, which will likely slow the progression of large action models more than anticipated. Investors should also be prepared for companies and startups that are more focused on selling the idea of a product rather than the product itself.
Given such pitfalls and dangers of marketing bias, executives and investors need to cooperate with global technology partners in order to identify valid innovative solutions and select the best fit for their enterprises.